
Team: The Outliers
Real-time Speech Emotion Recognition
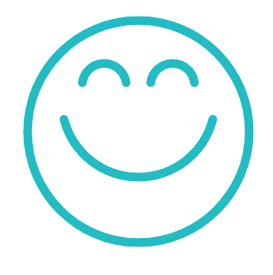
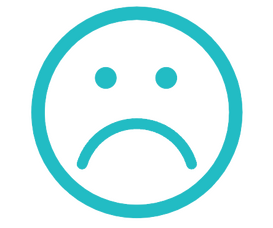
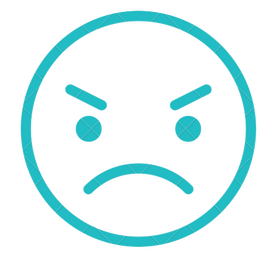
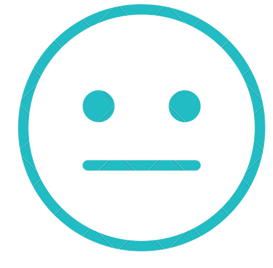
Our project builds conversational analytics & AI solutions that can analyze real-world speech and provide insights from text or audio data for Seasalt.ai.We implemented multiple Machine Learning models and found Convolution Neural Networks(CNN) to have improved the accuracy by around 67% which is the best-performing model.This model identifies the emotions from audio data clips to improve conversational analytics in Seasalt.ai's product SeaMeet to improve customer service and eliminate the feedback loop.
Opportunity
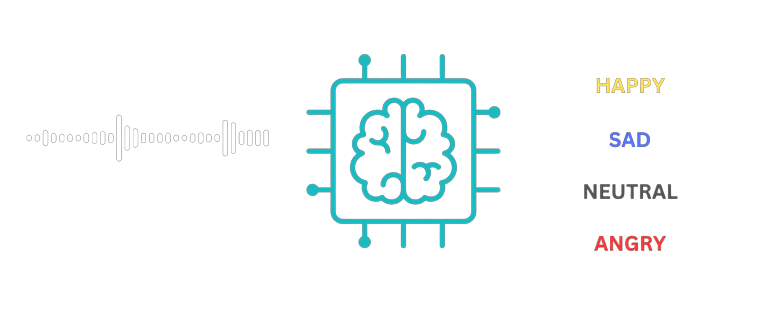
Seasalt.ai has a baseline “Speech Emotion Recognition Model” built on labeled audio data. However, the model has a baseline accuracy of 30% and we intend to improve that accuracy so that SeaMeet can detect emotions from audio with certainty.
Goal
We aim to accurately predict human emotion through machine learning and eventually help improve customer service and the quality of sales communication.
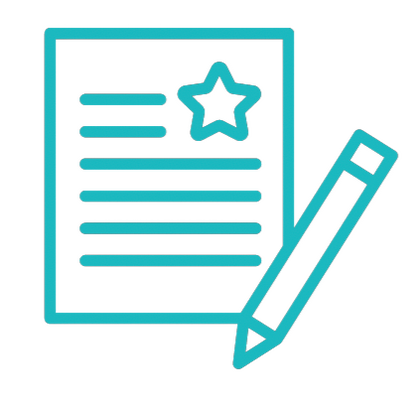
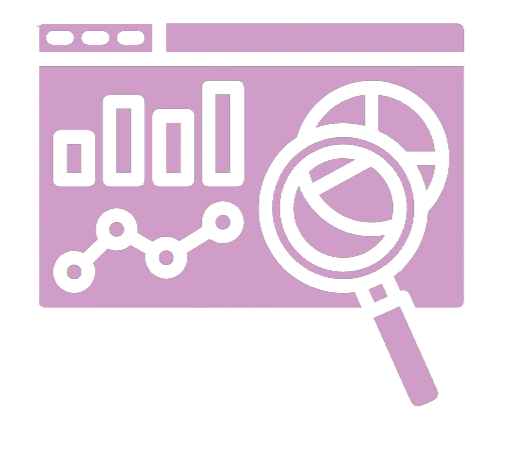
Impact
Improvements to the current system are being done by training the model on audio data that most closely resembles Seasal.ai's current data. By implementing complex machine learning modeling techniques, we have achieved an increase of 67% in the accuracy.
Methodology
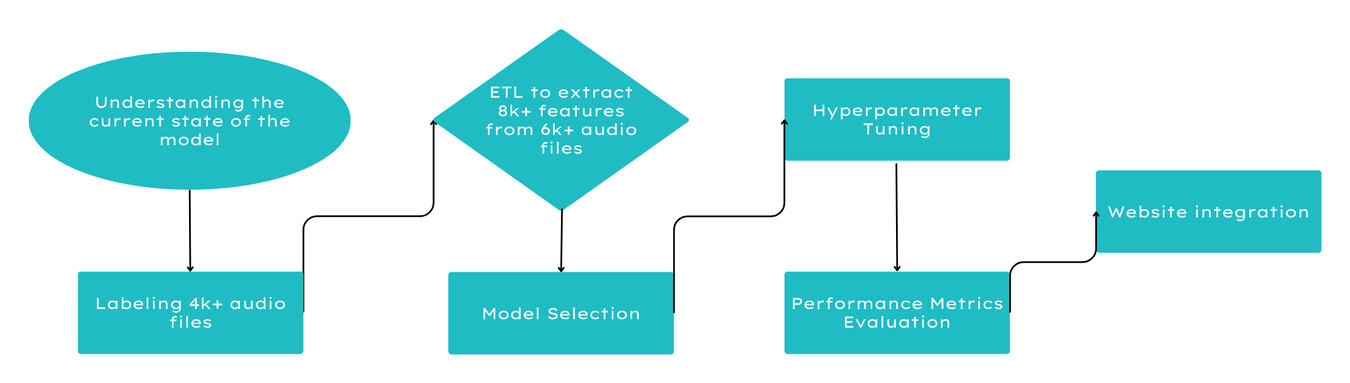
Our Work
This web application is built to compare and contrast different Emotion Model outputs. It can live record or upload pre-recorded audio along with 5 model variations to select from.
Included is an About page containing model and usage information.
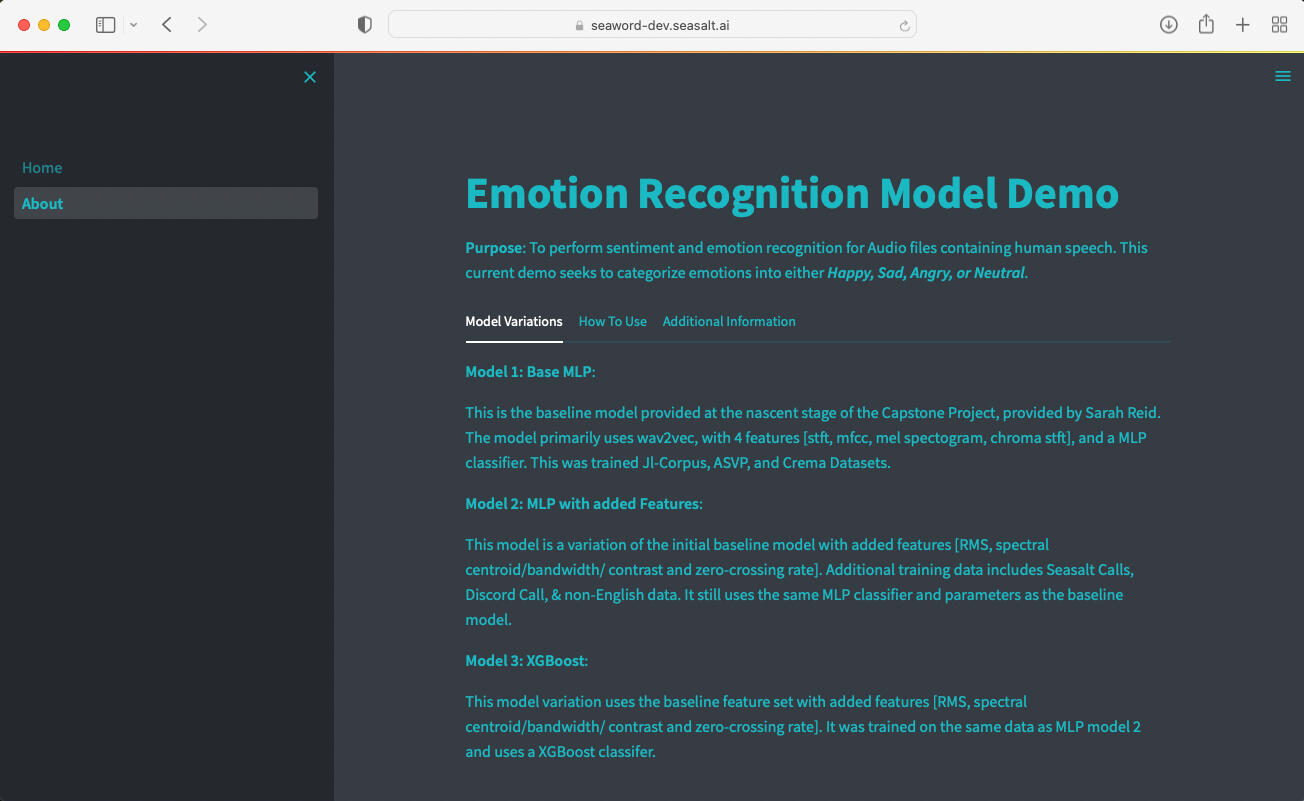
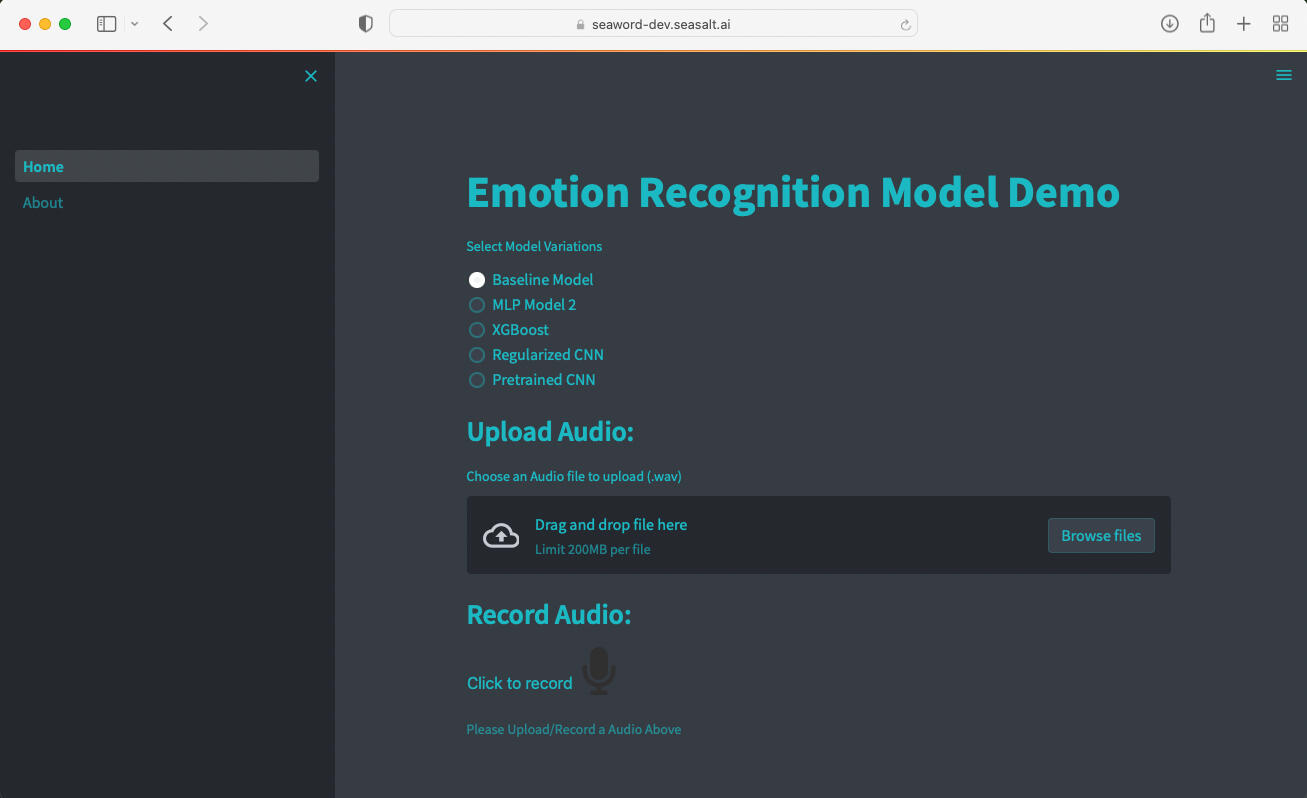
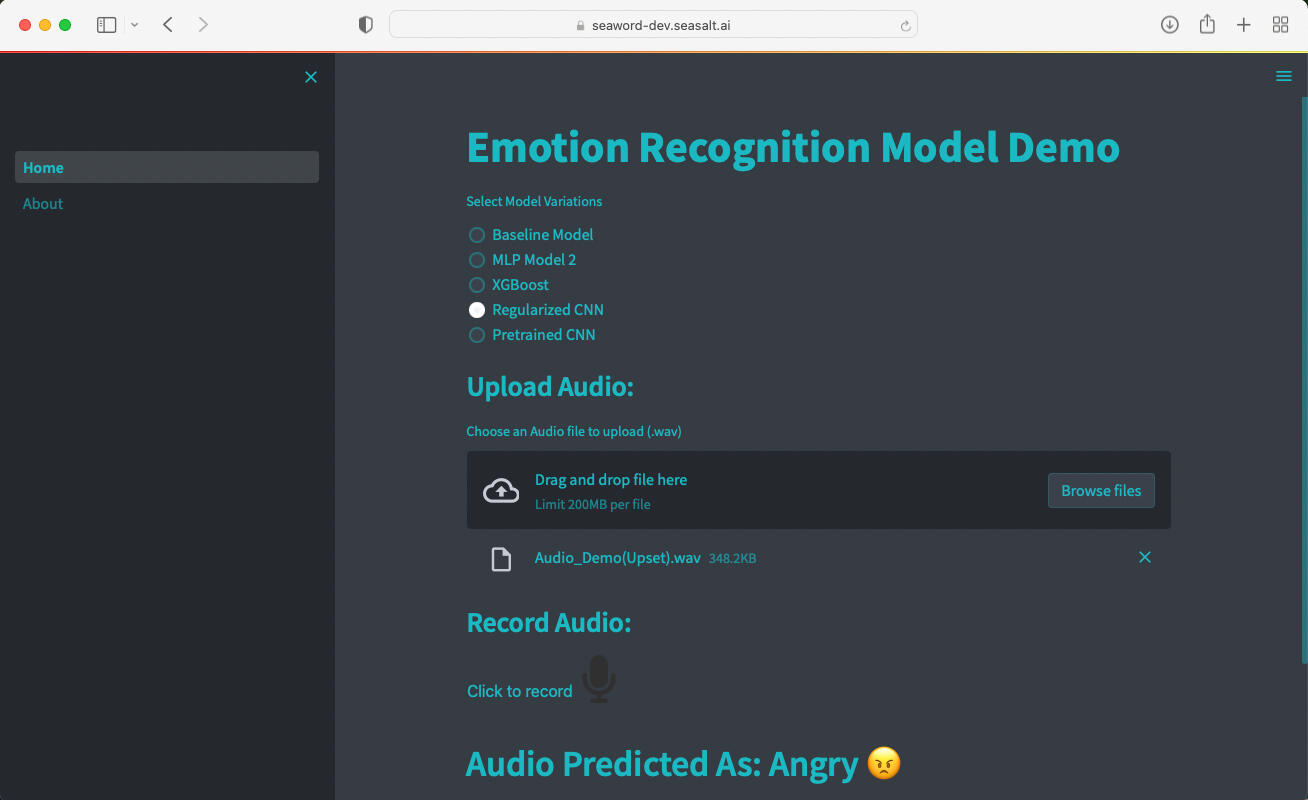
Meet the team
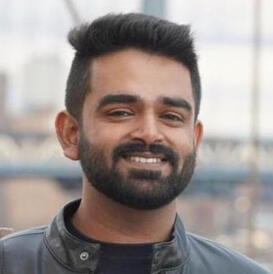
Nayan Kaushal
Data Scientist
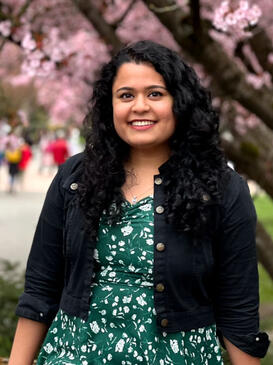
Punya Shetty
Full Stack Developer
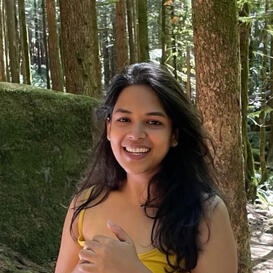
Rakshitha KN
Product Manager
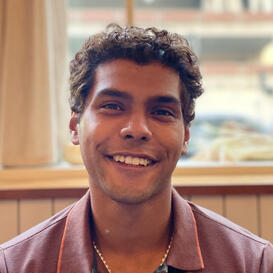
Walker Azam
Bioinformatician